AHA FIT Newsletter
FIT-Led Publications Corner: A Conversation with Dr. Evangelos Oikonomou
Zakaria Almuwaqqat, MD
Cardiology Fellow, PGY-6
Division of Cardiology, Emory School of Medicine
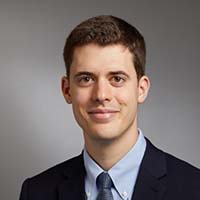
Evangelos (Evan) Oikonomou is a clinical fellow in cardiology and a member of the ABIM Physician-Scientist Research Pathway at Yale School of Medicine. He is a native of Athens, Greece where he also completed his medical school training at the University of Athens. Following his graduation, Evangelos moved to the United Kingdom to pursue a Ph.D. (D.Phil.) degree at the Radcliffe Department of Medicine, University of Oxford. During the course of his project entitled "Non-invasive phenotyping of perivascular adipose tissue for cardiovascular disease diagnosis and risk stratification", he developed machine learning-based non-invasive imaging approaches to better characterize the role of perivascular inflammation in atherosclerosis. As a physician-scientist, Evangelos is interested in applying machine learning approaches using multidimensional data derived from non-invasive imaging to individualize cardiovascular disease diagnosis and treatment.
Zakaria: Can you describe your journey in becoming a cardiology fellow and what your specific clinical/research interests are?
Evangelos: My journey towards becoming a cardiology fellow has been shaped by the wonderful mentors that I have had the privilege to meet and work with. Following my medical school graduation from the University of Athens in Greece, I moved to the United Kingdom to pursue a Ph.D. in Cardiovascular Science at the University of Oxford, U.K. There I joined Dr. Charalambos Antoniades’ lab and studied the biological interplay of perivascular adipose tissue with coronary atherosclerosis. Guided by our observations in the laboratory, we used machine learning approaches to identify phenotypic signatures of perivascular fat on non-invasive cardiac imaging to flag high-risk patients, improve the risk stratification of coronary artery disease, and prediction of adverse cardiovascular events. Through this process, I developed an interest in the field of biomedical data science and machine learning. I then moved to Yale, where I joined the Physician-Scientist Research Pathway, completed my internal medicine residency and I am now pursuing my clinical fellowship in Cardiology. I also joined the Cardiovascular Data Science (CarDS) lab, led by Dr. Rohan Khera, and began working on machine learning applications focusing on the personalized interpretation of clinical trials.
Zakaria: Would you briefly take us through your research paper that was published in European Heart Journal?
Evangelos: In our research paper, we tried to address a common clinical question that we all face in our daily clinical practice. Current guidelines recommend various alternative approaches in the non-invasive investigation of a patient presenting with stable chest pain. Building on the results of the PROMISE (Prospective Multicenter Imaging Study for Evaluation of Chest Pain) trial which demonstrated no significant difference in prospective outcomes between patients randomized to anatomical evaluation using coronary computed tomography angiography (CCTA) and functional stress testing, we utilized a machine learning-guided approach to identify whether specific patient phenotypes derive a differential benefit from either of the two strategies. To this end, we constructed a computational phenomap of the baseline patient phenotypes and developed an algorithm to look within each patient’s unique phenotypic neighborhood and explore which strategy was associated with better outcomes in a group of patients who were phenotypically most similar to our index patient. We repeated this process for each one of the 10,003 patients in the study and then trained a machine-learning algorithm to learn the observed treatment effect heterogeneity that we had uncovered. We named the model ASSIST (Anatomical versus Stress testing decision Support Tool) and made it available online for further use. Interestingly, when applying this algorithm to an external population, we were able to reliably identify patient groups that had improved cardiovascular outcomes when undergoing a diagnostic strategy that was concordant with the one proposed by our model.
Zakaria: How will publishing this article play a role in your next steps/future goals?
Evangelos: I have always been interested in precision medicine through deep phenotyping of biomedical images and data. Since this paper, we have been working on refining the method to enable broader applicability to a range of clinical trial designs as well as observational data. I am excited to be working as part of a multidisciplinary team along with computer scientists, data scientists, and fellow clinician-investigators to tackle big cardiovascular questions using a range of skillsets and expertise.
Zakaria: What advice would you give to other FITs thinking about using machine learning algorithms for cardiovascular research?
Evangelos: Machine learning algorithms are a powerful tool in cardiovascular research, however, they should be used wisely and guided by clinical and scientific reasoning. For any FITs who are interested in exploring the field, some wonderful online resources and textbooks can serve as a primer and introduction to the theoretical basis of machine learning and the basics of the most popular programming languages (Python, R). Most importantly, however, I would encourage them to identify mentors in their institutions who are experts in data science and machine learning and engage in clinically oriented projects, since the best way to learn is through real-world problem-solving.
Thanks to Dr. Evangelos Oikonomou for providing this perspective regarding his research journey and congratulations on winning first place in the Northwestern Cardiovascular Young Investigators’ Competition!